introduction
Before we dive into the details of Machine Learning (ML), it's crucial to understand that ML is a branch of Artificial Intelligence (AI). Often, the two terms are confused, but while AI encompasses a wide field that seeks to replicate human intelligence, ML focuses specifically on developing algorithms that allow machines to learn from data and make decisions without explicit human intervention.

In this blog, we will explain step by step how Machine Learning works, from the initial capture of data to the implementation of models in production. This knowledge is critical to understanding how organizations can automate complex tasks and make decisions based on data.
Machine Learning vs. Deep Learning
- Machine Learning (ML): Machine Learning is a branch of Artificial Intelligence that focuses on the development of algorithms and models that learn patterns from data and make predictions or decisions without explicit human intervention. ML models can be supervised, unsupervised, or reinforcement, depending on how they learn from data.
- Deep Learning: Deep Learning is a sub-area of Machine Learning that uses deep neural networks to learn hierarchical representations of data. It's especially useful for complex image, sound, and text processing problems, where patterns are difficult to capture with traditional ML methods.
Now, if we want to build a solution that works properly, we must always follow the following steps:
1. ETL process in Machine Learning
To build an effective Machine Learning solution, it is essential to follow a structured Extraction, Transformation and Load (ETL) process. This process ensures that the data is properly prepared before applying ML models, maximizing the accuracy and relevance of the predictions.
The ETL process is crucial because it ensures that the data is clean, structured, and ready for analysis. Here we explain each step of the process:
1.1 Data Capture/Extraction: Data capture is the first critical step in the Machine Learning process. Without quality and properly structured data, any model that is built may lack precision and relevance. Key methods and considerations for acquiring reliable data are explored here.
- Data is the fuel of Machine Learning. Every decision made by an ML model is based on the data it was trained and tested with. Therefore, the quality and quantity of the data are essential for the accuracy and generalization of the model.
- The data can come from various sources, such as transactional databases, event logs, CSV files, web APIs, IoT sensors, and others. It is crucial to select sources that are relevant and complete for the problem to be solved.
1.2 Data transformation: Once the data is captured, it needs to be transformed to properly prepare it for analysis. The transformation includes:
- Data Cleaning: Eliminate null values, correct errors and standardize formats to ensure data consistency and quality.
- Standardization and Standardization: Adjust the data so that it is on a uniform scale, which facilitates analysis and improves model performance.
- Feature Engineering: Create new variables or characteristics based on existing data to improve the predictive capacity of the model.
1.3 Loading the data: Once the data is transformed, it is loaded into a format suitable for use in Machine Learning models. This process involves:
- Integration with ML Platforms: Ensure that the prepared data is properly integrated with the ML platforms and tools used to build and train models.
- Data Validation: Verify the integrity and consistency of the uploaded data to avoid errors during analysis and modeling.
It is important to understand that the success of the ETL process in Machine Learning depends not only on the technology used, but also on a deep understanding of the problem domain and the data.
2. Model Selection:
Once the data has been successfully captured, transformed and loaded, the next critical step in the Machine Learning process is selecting the right model. The choice of model depends on the type of problem we are trying to solve and the nature of the available data.2.1 Types of Learning in Machine LearningMachine Learning is subdivided into different types of learning, each suitable for different tasks and types of data:
2.1 Supervised Learning: In supervised learning, models learn from labeled data that contains the correct answer. For example, in image classification, images labeled “dog” or “cat” are provided, and the model learns to predict the correct label for new images. Some common types of supervised learning models include:
Linear Regression:
- Use: It predicts continuous numerical values based on independent variables.
- Explanation: Suitable when you want to establish a linear relationship between variables, for example, predicting the price of a house based on characteristics such as size or location.
Logistic Regression:
- Use: Binary classification predicts the probability that an observation belongs to a class.
- Explanation: Ideal for problems where events need to be predicted, such as whether an email is spam or not, based on characteristics such as keywords and characteristics of the email.
Decision Trees:
- Use: Classification, or regression, divides data into subsets based on characteristics to make predictions.
- Explanation: Useful when you want to understand how decisions are made based on specific characteristics, such as predicting credit risk based on income, credit history, etc.
Random Forest:
- Use: Decision tree improvement, uses multiple trees to improve accuracy and avoid overadjustment.
- Explanation: Suitable when robust and accurate prediction is needed, combining the prediction of several trees to reduce the risk of errors due to bias or data variability.
Support Vector Machines (SVM):
- Use: Classification or regression, find the optimal hyperplane that best separates classes.
- Explanation: Ideal when you need to find a clear decision boundary between classes in high-dimensional data, such as classifying images or text data.
Each type of model has its advantages and disadvantages, and choosing the right model depends on the specific problem you're trying to solve, the nature of your data, and your prediction objectives.
2.2 Unsupervised Learning: In unsupervised learning, models are primarily used to discover hidden patterns or structures within data without explicit labels. Here are some common types of unsupervised models and their applications:
Clustering:
- Use: Grouping similar data into discrete sets.
- Explanation: Algorithms such as k-means, DBSCAN, and hierarchical clustering make it possible to identify natural groups within unlabeled data, such as segmenting customers according to purchasing behavior or grouping documents according to themes.
Association Analysis:
- Use: Identification of frequent patterns or associations between variables.
- Explanation: Applied in data mining to discover relationships between elements, such as product recommendations based on historical user purchases.
Dimensionality Reduction:
- Use: Simplification of data while keeping as much information as possible.
- Explanation: Methods such as t-SNE or MDS are useful for visualizing complex data in smaller spaces, preserving important relationships between points.
Anomaly Detection:
- Use: Identifying unusual observations or outliers in the data.
- Explanation: Important in fraud detection, predictive maintenance, or any case where anomalous data may indicate problems or unexpected behavior.
Matrix Decomposition:
- Use: Matrix factorization to identify latent structures.
- Explanation: It is used in recommendation systems and social network analysis to discover underlying patterns in large sets of matrix data.

2.3 Reinforcement Learning: In reinforcement learning, agents learn through trial and error, receiving rewards or punishments based on their actions. This is used in applications such as games and robotics, where agents learn through interaction with the environment. Here are some models:
Q-Learning:
- Use: Learning optimal policies in discrete environments.
- Explanation: The agent learns to make sequential decisions by maximizing the accumulated reward through the iterative update of the Q function, which estimates the expected value of taking an action in a specific state.
Exploration vs. Exploitation:
- Use: Balance between making known decisions and exploring new actions to discover better policies.
- Explanation: It's crucial in reinforcement learning to avoid getting stuck in suboptimal policies and discovering actions that can lead to greater long-term rewards.
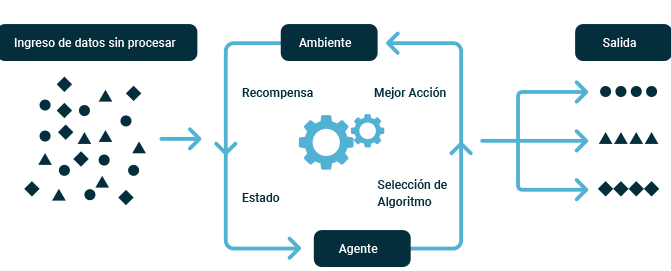
3. Evaluation of the Model
Once a Machine Learning model has been selected and trained, it is essential to evaluate its performance before implementing it in production. The evaluation provides crucial information about the model's ability to generalize to new data and its accuracy in the specific task for which it was designed. In this section, we explore different techniques and metrics used to evaluate Machine Learning models.
3.1 Evaluation Metrics
- Confusion Matrix: The confusion matrix is a fundamental tool for evaluating the performance of a classification model. It allows us to visualize the number of correct and incorrect predictions in each class, making it easier to identify errors such as false positives and false negatives.
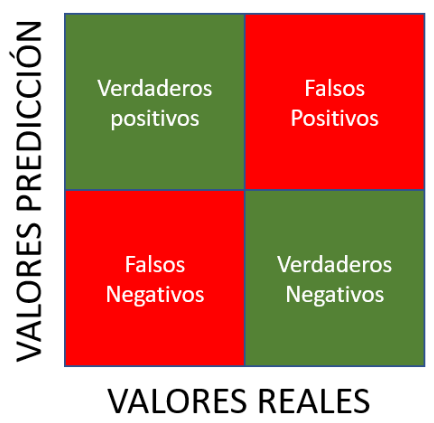
- Accuracy, Recall and F1-Score: These metrics are common in classification problems and provide a detailed understanding of model performance in terms of accuracy (how many positive predictions are correct), recall (how many of the true positives the model detected) and F1-score (a combined measure of accuracy and recall).
- ROC Curve and Area Under the Curve (AUC-ROC): The ROC (Receiver Operating Characteristic) curve is useful for evaluating binary classification models, showing the relationship between the true positive rate and the false positive rate across different decision thresholds. The AUC-ROC provides an aggregated measure of model performance.
3.2 Hyperparameter Optimization
- Hyperparameters are adjustable settings that are not learned directly from the model training process. Optimizing these hyperparameters can significantly improve model performance. Common techniques include exhaustive search (Grid Search) and random search (Random Search) to find the optimal combination of hyperparameters.
3.3 Cross-Validation
- Cross-validation is a technique for evaluating the performance of a model using multiple subsets of training and test data. This helps mitigate the risk of overfitting and provides a more robust evaluation of model performance on unseen data.
3.4 Interpretation of Results
- It is crucial to interpret the results of the evaluation metrics and the visualizations obtained during the model evaluation process. This allows the model to be adjusted if necessary, to understand its strengths and weaknesses, and to make informed decisions about its implementation and continuous improvement.
4. Putting the Model into Production
Once a well-performing Machine Learning model has been trained and evaluated, the next crucial step is to implement it in a production environment for use in real applications. This phase involves several processes and considerations to ensure that the model works effectively, efficiently and in a scalable manner.
4.1 Preparing for Implementation
- Optimization and Compaction of the Model: Before implementation, it is common to optimize the model to reduce its size and complexity, improving computational efficiency and accelerating inferences. Techniques such as model quantization and weight pruning can be used for this purpose.
- Integration with Existing Infrastructure: The model must be integrated with the existing software and hardware infrastructure in the production environment. This can include database management systems, APIs for communicating with other applications, and data storage and processing services.
4.2 Version Management and Quality Control
- Version Management: It is crucial to implement a robust version management system to track changes to the model and ensure that updates are deployed in a controlled and reversibly manner. Tools such as Git and model-specific controllers can be used for this purpose.
- Testing and Validation in a Production Environment: Before launching the model into production, extensive tests must be carried out to ensure that it works properly in different scenarios and load conditions. Cross-validation and stress testing are useful for identifying potential problems and optimizing performance.
4.3 Continuous Monitoring and Maintenance
- Performance Monitoring: Once in production, it's crucial to continuously monitor the model's performance to detect deviations in accuracy or efficiency. This may involve monitoring performance metrics in real time and setting up automatic alerts for potential problems.
- Maintenance and Update: Machine Learning models are dynamic and may require periodic adjustments to maintain their accuracy and relevance. Maintenance includes updating training data, retraining the model with new data, and optimizing hyperparameters as needed.
4.4 Scalability and Security
- Scalability: The design of the implementation architecture must consider the ability to scale the model to handle an increase in workload or expansion of the system's reach. Using containers such as Docker and deploying them on automatic scaling platforms such as Kubernetes are common practices.
- Safety: The security of models in production is crucial to protect sensitive data and ensure system integrity. This can include practices such as data encryption, access management, and the implementation of security measures in APIs and access points to the model.
The production of a Machine Learning model is a complex process that requires careful planning and execution. Following best practices and considering the specific needs of the environment, it is ensured that the model can effectively and sustainably generate value in the real world.
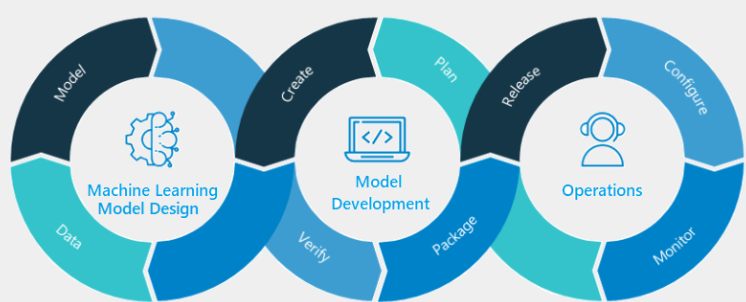
Glossary:
Bias:
- In Machine Learning, bias refers to the difference between the model's prediction and the true value being tried to predict. A high bias indicates that the model cannot capture the underlying relationship between the data, which can lead to inaccurate and non-generalizable predictions.
Hyperplane:
- A hyperplane is a generalization of the concept of a plane or line in higher-dimensional spaces. In Machine Learning, especially in classification algorithms such as Support Vector Machines (SVM), a hyperplane is used as a decision frontier that separates different classes in the feature space.
Clustering:
- Clustering is the process of grouping a set of objects in such a way that objects in the same group (or cluster) are more similar to each other than to those of other groups. It's a common method in unsupervised learning to explore hidden patterns and structures in data.
K-means:
- K-means is a clustering algorithm that groups data into K groups based on their characteristics. It works by assigning data points to the group whose centroid (midpoint) is closest to them, iteratively optimizing the position of the centroids until they converge into a solution.
DBSCAN:
- DBSCAN (Density-Based Spatial Clustering of Applications with Noise) is a clustering algorithm that groups data points into clusters based on the local density of the points. It is able to identify clusters of arbitrary shapes and efficiently handle data with noise and outliers.
T-SNE (T-distributed Stochastic Neighbor Embedding):
- T-SNE is a nonlinear dimensionality reduction technique used primarily for the visualization of high-dimensional data sets. It focuses on preserving local relationships between data points, allowing us to reveal complex structures in data in a smaller space.
MDS (Multidimensional Scaling):
- MDS is a statistical analysis technique used to visualize the similarity between objects. It transforms the similarities between data points into distances in a smaller space, preserving the relationships between them as much as possible.
Agent:
- In the context of reinforcement learning, an agent is an entity that interacts with an environment with the objective of maximizing an accumulated reward over time. The agent makes decisions based on his learned action policy and observations of the environment.
Quantization:
- In the context of neural networks or data analysis, quantization refers to the process of reducing the number of different values of a variable. It may involve reducing numerical precision to simplify calculations or clustering similar values to simplify models.
Pruning:
- In the context of decision trees and other tree-based models, pruning refers to the process of removing unwanted or irrelevant sections of a tree to improve its accuracy and generalizability. Pruning can be pre-pruning (before full construction of the tree) or post-pruning (after construction).
Grid Search:
- Grid Search is a technique for finding the best hyperparameters for a Machine Learning model. It comprehensively evaluates the hyperparameter combinations specified in a predefined grid, using cross-validation to determine which combination provides the best performance.
Random Search:
- Random Search is a strategy for hyperparameter optimization that selects random combinations of hyperparameters to evaluate model performance. Unlike Grid Search, it doesn't evaluate all possible combinations, which can be more computationally efficient in large or complex search spaces.
Ready to implement Machine Learning solutions in your projects?
At Kranio, we have experts in artificial intelligence who will help you develop and implement Machine Learning models adapted to the needs of your business. Contact us and discover how we can drive the digital transformation of your company through machine learning.